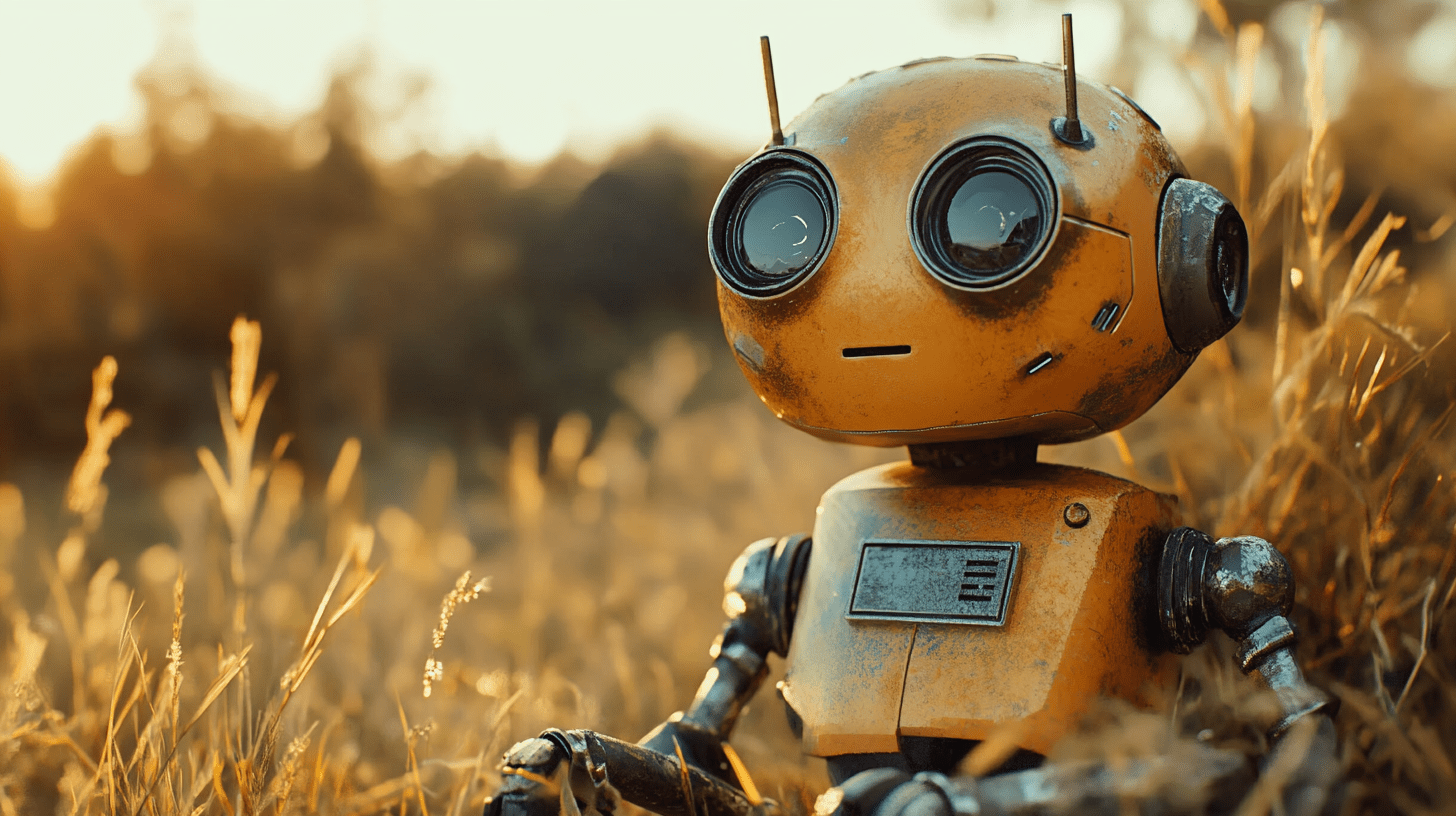
5 Causes Why Conventional Machine Studying is Alive and Nicely within the Age of LLMs
Picture by Editor | Midjourney
These days, everybody throughout AI and associated communities talks about generative AI fashions, notably the massive language fashions (LLMs) behind widespread functions like ChatGPT, as if they’ve fully taken over the sphere of machine studying. Nonetheless, conventional AI methods, together with these based mostly on classical machine studying fashions and algorithms, are removed from extinct. They continue to be important for a lot of real-world functions attributable to their skill to effectively resolve particular issues and their domain-specific benefits when they’re correctly skilled.
This text presents 5 explanation why conventional machine studying options are right here to remain.
1. Conventional Machine Studying Fashions are Nonetheless the Proper Solution to Remedy Some Frequent Knowledge Issues
For those who want one most important purpose why conventional machine studying is right here to remain, let or not it’s this one. Have you ever ever heard the saying, “utilizing a sledgehammer to crack a nut”? This analogy emphasizes the mismatch that happens when an excessively advanced device is utilized to a easy downside. LLMs have revolutionized the best way we work together with AI methods utilizing pure language — whether or not for asking advanced questions, translating textual content, or producing lifelike content material. Nonetheless, LLMs are primarily generative fashions: they concentrate on producing high-quality, artistic content material in response to person queries—a talent developed by coaching on huge quantities of textual content information.
But, lengthy earlier than generative AI, machine studying fashions had been designed to sort out predictive and descriptive duties: classifying clients or photographs, estimating costs or gross sales, performing buyer segmentation, and extra. These predictive issues stay important in each every day life and enterprise operations. Regardless of the astonishing capabilities of LLMs, conventional machine studying fashions proceed to excel in these domains and are unlikely to get replaced anytime quickly.
Whereas the above is likely to be crucial purpose for conventional machine studying to stick with us, 4 extra particular causes additional assist help this declare.
2. Effectivity and Value-Effectiveness
Classical machine studying fashions are steadily computationally cheaper than large LLMs with billions of trainable parameters; the previous requires a lot fewer “gears and switches” to do their meant job successfully. Additional, they require much less information and computing energy than LLMs, being extra appropriate in resource-constrained settings.
Conventional fashions usually additionally require solely a fraction of the time to coach in comparison with LLMs. Whereas some conventional fashions could be skilled in minutes or a couple of hours on customary {hardware}, LLMs would possibly want days of coaching on specialised GPU clusters. As well as, their smaller reminiscence footprint and decrease vitality consumption translate straight into lowered cloud and operational prices. This effectivity makes conventional fashions particularly appropriate for real-time functions and edge computing environments the place computational assets are restricted.
3. Explainability and Interpretability
Some machine studying fashions like linear regressors and resolution bushes present clear decision-making insights, facilitating an understanding of why they made the predictions they made for enter information examples. This is essential for dependable predictive methods deployed in regulated industries like healthcare and finance.
Furthermore, strategies resembling SHAP (SHapley Additive exPlanations) and LIME (Native Interpretable Mannequin-agnostic Explanations) additional improve the transparency of those fashions by quantifying the affect of every function on the prediction end result. This degree of detailed perception is particularly useful in industries with strict regulatory oversight, the place understanding the reasoning behind a mannequin’s prediction is essential for auditing and compliance. Moreover, a number of case research have demonstrated that extra interpretable fashions can enhance each belief and adoption amongst end-users.
4. Specialization and Exploitation of Structured Knowledge
LLMs are skilled to turn into glorious in understanding and dealing with unstructured information like textual content, however conventional machine studying fashions don’t have any rival in managing purely structured information issues like fraud detection, gross sales or worth forecasting, and optimization. They’re skilled by understanding advanced patterns amongst information variables and inferring relationships between inputs or predictor attributes, and goal outputs to foretell or estimate.
Moreover, the power to carry out intricate function engineering permits practitioners to customise fashions to satisfy the distinctive challenges of structured information. Strategies resembling gradient boosting — applied in algorithms like XGBoost or LightGBM — have turn into business requirements for dealing with tabular information attributable to their excessive robustness and accuracy. The tip-to-end course of from information preprocessing to mannequin analysis could be optimized in conventional machine studying workflows, making certain superior efficiency on key metrics resembling precision, recall, and F1 rating.
5. Simpler Deployment and Upkeep
Whereas LLMs are extraordinarily helpful in quite a lot of language-related use circumstances, coaching, deploying, and sustaining them is advanced in comparison with typical machine studying fashions, which are usually simpler to construct and operationalize with out essentially demanding a large infrastructure. This makes them sensible for myriad enterprise functions.
As well as, conventional fashions profit from easier manufacturing pipelines and could be simply built-in into present methods utilizing light-weight frameworks like scikit-learn. This ease of integration facilitates faster upkeep cycles and permits groups to replace or retrain fashions with minimal disruption. Moreover, their decrease useful resource calls for make them ultimate candidates for deployment in edge computing environments, the place processing energy is proscribed.
Wrapping Up
Conventional machine studying stays indispensable in at the moment’s AI panorama, as now we have argued by 5 key causes, from effectivity and interpretability to specialization with structured information and ease of deployment. Conventional strategies are nonetheless the optimum alternative for a lot of functions. Do not forget that the most effective device is the one that matches the duty at hand, so gravitating towards complexity for complexity’s sake is rarely an optimum strategy to problem-solving.
Source link