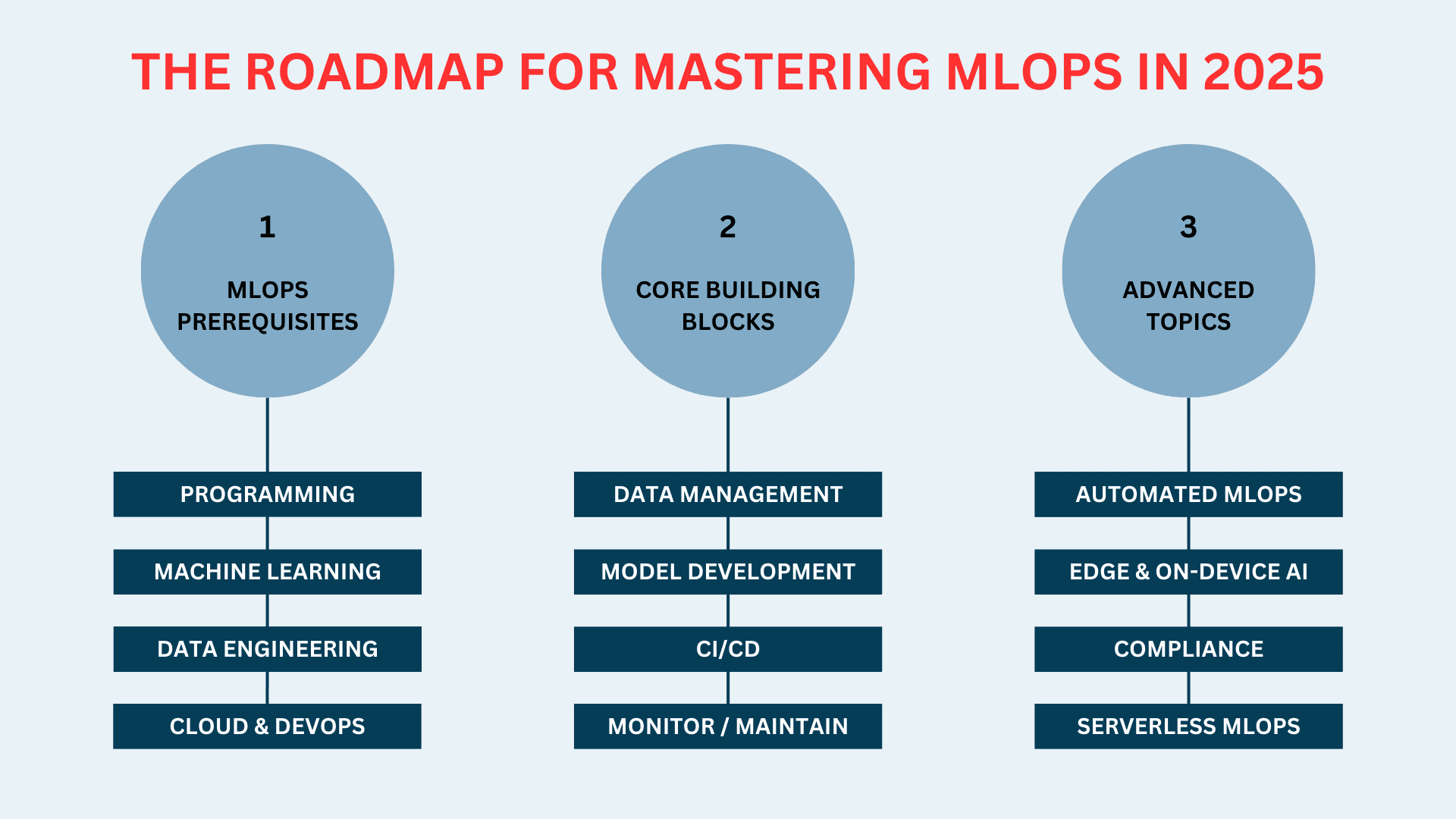
The Roadmap for Mastering MLOps in 2025
Picture by Editor | Canva
Organizations more and more undertake machine studying options into their each day operations and long-term methods, and, because of this, the necessity for efficient requirements for deploying and sustaining machine studying techniques has grow to be vital. MLOps (brief for machine studying operations) arose to satisfy these wants. It encompasses a collection of practices that mix machine studying modeling, software program engineering, and knowledge engineering throughout your complete machine studying system lifecycle.
If you’re eager on venturing into the realm of MLOps in 2025 and not sure of the place to start out, this text highlights and places collectively its constructing blocks and newest traits, each essential to achieve understanding of the present MLOps panorama.
Understanding MLOps
The main focus of MLOps is streamlining the method of bringing skilled machine studying fashions — like picture classifiers, gross sales predictors, rainfall forecasting fashions, and so forth — from a growth setting into real-world manufacturing environments. MLOps will be seen as a lifecycle consisting of a number of phases:
- Knowledge assortment and preprocessing
- Mannequin coaching
- Mannequin deployment
- System monitoring and governance
MLOps integrates rules from a well-established set of software program growth practices: DevOps. Thus, it ensures that machine studying fashions grow to be reproducible, scalable, and simpler to take care of.
Step 1: Conditions
Earlier than diving into MLOps, be sure to get aware of prerequisite ideas and disciplines that type the muse for this subject. These embrace programming, machine studying fundamentals, and DevOps rules.
- Python Programming Language and Bash scripting: these are two software program growth necessities these days, and their significance additionally extends to frequent DevOps and MLOps processes like automating workflows, managing infrastructure, and defining interactions between MLOps instruments and elements. Study extra about Python here and Bash here.
- Machine Studying Fundamentals: this one could sound apparent, however efficiently mastering MLOps implies turning into aware of machine learning algorithms and important processes like model training, utilizing evaluation metrics, and getting acquainted with in style machine studying growth frameworks like scikit-learn, TensorFlow, or PyTorch.
- Knowledge Engineering Fundamentals: study the basics of knowledge wrangling, ETL (Extract-Transform-Load) pipelines, and get used to working with structured (tabular) and unstructured knowledge like textual content or photographs.
- DevOps and Cloud: place deal with understanding processes and instruments like containerization (Docker), orchestration (Kubernetes), and cloud computing platforms (AWS, GCP, or Azure).
- Software program Engineering Greatest Practices: fine-tune your software engineering expertise, staying updated on model management (Git) and associated instruments, code testing, and modular programming.
Step 2: Core Constructing Blocks
According to the 4 lifecycle levels launched earlier, the core constructing blocks of MLOps you must find out about after you have acquired the prerequisite data, will be summarized into:
- Knowledge Administration: study knowledge versioning with instruments like DVC (Data Version Controlling), assimilate the rules for guaranteeing knowledge high quality, and implement function shops upon your datasets for enabling reusable inputs throughout ML fashions.
- Mannequin Improvement: deal with reproducibility, experiment tracking by utilizing instruments like MLflow, and make your self snug with mannequin versioning rules and greatest practices.
- Steady Integration and Steady Deployment (CI/CD): get aware of CI/CD pipelines for ML. Well-liked instruments for this embrace GitHub Actions, AWS Jenkins, or GitLab CI/CD.
- Monitoring and Upkeep: as soon as your ML model has been deployed to production, you’ll wish to discover ways to monitor its efficiency throughout the time, detect points like knowledge and idea drifts, and configure automated retraining workflows able to updating your fashions as soon as their efficiency degradation has surpassed a sure threshold, so it stays up-to-date and aligned with the present knowledge it receives.
Step 3: Rising Traits and Superior Subjects
At this level, you must have gained a stable understanding of MLOps ideas, rules, and most established strategies. To adapt to the ever-evolving nature of contemporary ML and AI techniques, MLOps additionally retains evolving with new traits and strategies. It’s time to get aware of cutting-edge developments:
- Automated MLOps: discover how the most recent paradigms like AutoML and AI-driven workflow automation can play a vital function in enhancing deployment effectivity.
- Edge AI and On-Machine ML: examine and perceive methods to deploy and keep ML fashions on edge devices like handheld techniques utilizing frameworks like TensorFlow Lite, ONNX, or NVIDIA Jetson.
- Mannequin Explainability (XAI) and Accountable AI: study the significance of interpretability in ML techniques and get aware of explainable AI instruments like SHAP and LIME to make sure transparency and equity in your deployed fashions.
- Safety and Compliance: ML techniques closely depend on real-world knowledge, typically of delicate nature. Research newest data privacy regulations (GDPR, CCPA) and MLOps safety greatest practices to guard your fashions and the info they eat.
- Serverless MLOps: one of many newest MLOps traits, it examines methods to deploy ML fashions utilizing serverless technologies within the cloud like AWS Lambda or Google Cloud Features.
Wrapping Up
This text supplied a roadmap that can assist you outline your pathway to mastering MLOps, a key machine studying pattern that continues to achieve floor in 2025 and is anticipated to more and more permeate most organizations and companies throughout sectors, attributable to its capacity to streamline ML mannequin deployment, facilitate automation, assure scalability, and improve the reliability of ML-driven techniques.
The structured strategy of this roadmap guides by buying the prerequisite data to MLOps, acquainting with its core foundations, and venturing into the most recent and cutting-edge traits to achieve a sophisticated understanding of the subject.
Source link